For practitioners working with Salesforce's Agentforce, understanding how Data Cloud powers AI capabilities is crucial for building effective solutions. This guide explores the practical aspects of this integration and what it means for your day-to-day work with AI agents.
Understanding the Data Foundation
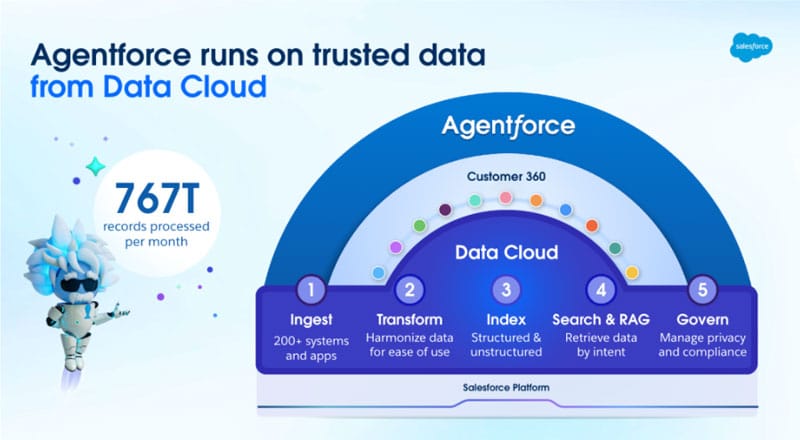
The diagram above illustrates how Agentforce operates through Data Cloud's five-stage pipeline. This architecture isn't just a theoretical framework—it's the foundation that determines how effectively your AI agents will perform in real-world scenarios.
The Five Stages: What They Mean in Practice
Stage 1: Ingestion
Working with over 200 systems and apps, the ingestion stage is your gateway to comprehensive data access. As a practitioner, this means you can pull data from virtually any source system without building custom integrations for each one. The real power lies in its ability to handle both structured and unstructured data seamlessly.
When starting a new project, begin by mapping out your key data sources. Focus particularly on systems containing customer interaction data—these will provide the richest context for your AI agents. Consider which data sources will add the most value to your specific use case, rather than trying to integrate everything at once.
Stage 2: Transform
Data transformation is where the magic of harmonization happens. This stage goes beyond simple data cleaning—it's about creating a cohesive data narrative that your AI agents can understand and use effectively. The transformation process ensures that data from different sources speaks the same language.
In practice, this means establishing consistent data models that make sense to your AI agents. For instance, when dealing with customer data from multiple systems, you'll want to ensure that customer identifiers, contact information, and interaction histories are standardized and properly linked.
Stage 3: Index
Indexing makes your data discoverable and usable. This stage is particularly crucial for practitioners because it determines how quickly and accurately your agents can access information. The indexing process handles both structured data (like database records) and unstructured content (such as emails and documents), creating a searchable knowledge base.
Pay special attention to how you structure your indexes. Well-designed indexing strategies significantly impact your agent's ability to find relevant information quickly. Consider the types of queries your agents will need to handle and ensure your indexing strategy supports these use cases.
Stage 4: Search & RAG
The Search & RAG (Retrieval Augmented Generation) stage is where your data becomes truly actionable. This capability combines traditional keyword search with semantic understanding, enabling your agents to find information based on context and intent, not just exact matches.
For practitioners, this means you can build agents that understand natural language queries and provide contextually relevant responses. When implementing search functionality, test with real-world scenarios and actual customer queries to ensure your agents retrieve appropriate information consistently.
Stage 5: Govern
Governance isn't just about compliance—it's about building trust. This stage ensures that your AI agents operate within appropriate boundaries, protecting sensitive data while maintaining functionality. Implement clear access controls and data retention policies, but also monitor how your agents use data to continually improve their performance.