Introduction
Large Language Models (LLMs) continue to push the boundaries of artificial intelligence, but their increasing size and computational demands present significant challenges. DeepSeek-V3, a new open-source model, offers a breakthrough by balancing high performance with cost-effective training. With a Mixture-of-Experts (MoE) architecture and innovative training methodologies, DeepSeek-V3 rivals leading closed-source models like GPT-4o while remaining accessible to the AI research community.
Key Features and Innovations
1. Mixture-of-Experts Architecture for Efficient Computation
DeepSeek-V3 employs a 671B-parameter MoE model, with only 37B parameters activated per token. This architecture optimizes computational efficiency, enabling high performance while significantly reducing the inference cost compared to dense models of similar scale.
2. Multi-Head Latent Attention (MLA)
Building on the advancements of DeepSeek-V2, MLA enhances inference efficiency by leveraging low-rank joint compression techniques. This results in a reduced Key-Value cache requirement without sacrificing model accuracy.
3. Auxiliary-Loss-Free Load Balancing
DeepSeek-V3 introduces an innovative load balancing strategy that eliminates the need for auxiliary loss, a common method in MoE training that can negatively impact model performance. Instead, a dynamic bias adjustment ensures even workload distribution among experts, improving training stability and efficiency.
4. Multi-Token Prediction (MTP) for Faster Training
Unlike traditional models that predict only the next token, DeepSeek-V3 trains on multiple future tokens at each step. This increases data efficiency and improves overall model performance, enabling better context understanding and speculative decoding for accelerated inference.
5. FP8 Mixed Precision Training for Cost Efficiency
DeepSeek-V3 pioneers the use of FP8 mixed precision training, reducing memory footprint and improving computational throughput. By implementing fine-grained quantization techniques, it maintains high numerical stability while cutting training costs.
Performance Benchmarks
DeepSeek-V3 sets new benchmarks across a variety of domains, including:
- Mathematical Reasoning: Achieves 90.2% accuracy on MATH-500, outperforming Qwen2.5 and LLaMA-3.1.
- Code Generation: Leads in HumanEval Pass@1 with 82.6%, surpassing major open-source models.
- General Knowledge: Excels in MMLU (88.5%) and GPQA-Diamond (59.1%), competing with top-tier closed-source models.
- Long-Context Understanding: Successfully processes up to 128K tokens, validated by the "Needle In A Haystack" test.
Real-World Applications
DeepSeek-V3’s capabilities make it a valuable tool across multiple domains:
- Enterprise AI Assistants: Its strong general reasoning and long-context capabilities make it suitable for business applications.
- Software Engineering: Leading performance in coding benchmarks positions it as a top choice for AI-assisted programming.
- Scientific Research: Robust mathematical and reasoning skills make it a powerful model for technical and academic applications
Cost-Effective Training
One of DeepSeek-V3’s standout achievements is its efficient training pipeline. Despite its size, the model was trained using only 2.788M H800 GPU hours, equating to approximately $5.576M in total costs—a fraction of what is typically required for similarly scaled models.
Future Directions
DeepSeek-AI plans to refine its architecture, further improving training efficiency and extending model capabilities beyond the Transformer framework. Future versions may explore infinite context length support and enhanced deep reasoning capabilities, moving closer to the vision of Artificial General Intelligence (AGI).
Conclusion
DeepSeek-V3 sets a new standard for open-source AI, proving that cutting-edge performance does not have to come with exorbitant computational costs. With its efficient MoE architecture, FP8 training, and groundbreaking techniques like Multi-Token Prediction, it represents a significant step forward in AI model development. Researchers and developers can explore DeepSeek-V3’s capabilities by accessing the model on GitHub.
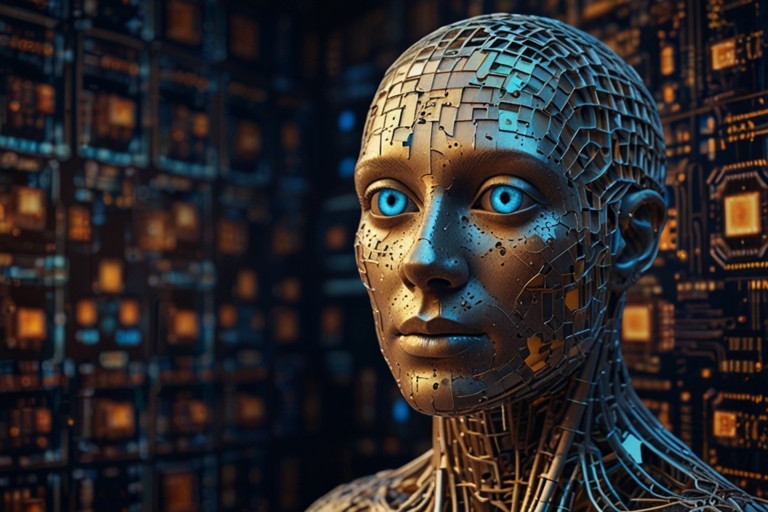